SPSS Mixed Model ANOVA is a powerful statistical tool used for analyzing complex datasets that involve both fixed and random effects. This method is particularly useful in experimental research where multiple measurements are taken from the same subjects, allowing researchers to account for variability within their data. By incorporating both types of effects, SPSS Mixed Model ANOVA provides a robust framework for understanding the relationships between variables and how they interact over time or across different conditions.
Researchers frequently encounter situations where traditional ANOVA does not suffice, especially in longitudinal studies or repeated measures designs. The mixed model approach offers a flexible solution by allowing for the inclusion of both fixed effects, which are consistent across all observations, and random effects, which vary among subjects or experimental units. This versatility makes SPSS Mixed Model ANOVA an essential tool in fields such as psychology, medicine, and social sciences, where the intricacies of human behavior and treatment responses often require more nuanced analysis.
In this article, we will delve into the intricacies of SPSS Mixed Model ANOVA, exploring its applications, advantages, and the step-by-step process for conducting this analysis in SPSS. Whether you are a seasoned researcher or a novice eager to learn, this guide will equip you with the knowledge needed to effectively utilize this powerful statistical technique.
What is SPSS Mixed Model ANOVA?
SPSS Mixed Model ANOVA is an advanced statistical method that combines the principles of Analysis of Variance (ANOVA) with mixed effects modeling. It is particularly beneficial when dealing with hierarchical or nested data structures. Unlike traditional ANOVA, which assumes independence of observations, mixed models allow for the correlation of repeated measures within subjects.
How Does SPSS Mixed Model ANOVA Work?
This method works by partitioning the total variability in the data into components attributable to different sources. The fixed effects represent the systematic influences on the dependent variable, while the random effects account for individual differences or variability among subjects. By modeling both fixed and random effects simultaneously, SPSS Mixed Model ANOVA provides a more accurate estimation of treatment effects and interactions.
Why Use SPSS Mixed Model ANOVA?
There are several compelling reasons to choose SPSS Mixed Model ANOVA for your analysis:
- Handles repeated measures and hierarchical data structures.
- Accounts for variability among subjects or experimental units.
- Provides more accurate estimates of fixed effects.
- Allows for complex designs, including unbalanced data.
When Should You Use SPSS Mixed Model ANOVA?
SPSS Mixed Model ANOVA is particularly useful in scenarios where:
- Data involves repeated measurements from the same subjects.
- There are multiple groups or treatments being compared.
- Random effects are expected to influence the outcome variable.
What are the Assumptions of SPSS Mixed Model ANOVA?
Before performing an SPSS Mixed Model ANOVA, it is essential to understand its underlying assumptions:
- The dependent variable should be continuous and normally distributed.
- Observations must be independent within and across groups.
- Random effects should be normally distributed.
- Homogeneity of variance across groups.
How to Conduct SPSS Mixed Model ANOVA?
Conducting SPSS Mixed Model ANOVA involves several steps:
- Open your dataset in SPSS.
- Select "Mixed Models" from the Analyze menu.
- Define your model by specifying fixed and random effects.
- Choose the appropriate covariance structure.
- Run the analysis and interpret the output.
Interpreting Results from SPSS Mixed Model ANOVA
After running the analysis, the output will provide several key statistics, including:
- Fixed effects estimates and significance levels.
- Random effects variance components.
- Model fit statistics.
It is crucial to interpret these results in the context of your research question and hypotheses, taking into account any interactions between variables that may have emerged.
What Are the Limitations of SPSS Mixed Model ANOVA?
While SPSS Mixed Model ANOVA is a powerful tool, it does have some limitations:
- Complexity in model specification can lead to misinterpretation.
- Requires a larger sample size for reliable estimates of random effects.
- Assumptions must be met to ensure valid results.
Conclusion: The Importance of SPSS Mixed Model ANOVA
In summary, SPSS Mixed Model ANOVA is a vital statistical technique for analyzing data with repeated measures and hierarchical structures. Its ability to account for both fixed and random effects makes it a versatile choice for researchers across various disciplines. By understanding its applications, assumptions, and execution, you can harness the power of SPSS Mixed Model ANOVA to gain deeper insights into your data and enhance your research outcomes.
You Might Also Like
Delightful Décor: Fake Popcorn String For Christmas TreeUnraveling The Mystery Behind The Fallout 3 Guy Yelling In The Alley
Understanding Semi Trailer Axle Replacement: A Comprehensive Guide
Unveiling The Depths Of Real Emotion Lyrics
Exploring The Enigmatic World Of Snape Art
Article Recommendations
- The Unfolding Drama Morning Joe Cast Fired
- Renowned Journalist Ted Koppel An Icon In American Broadcasting
- The Untold Story Of Kyle Dutcher Net Worth Biography Success And Achievements
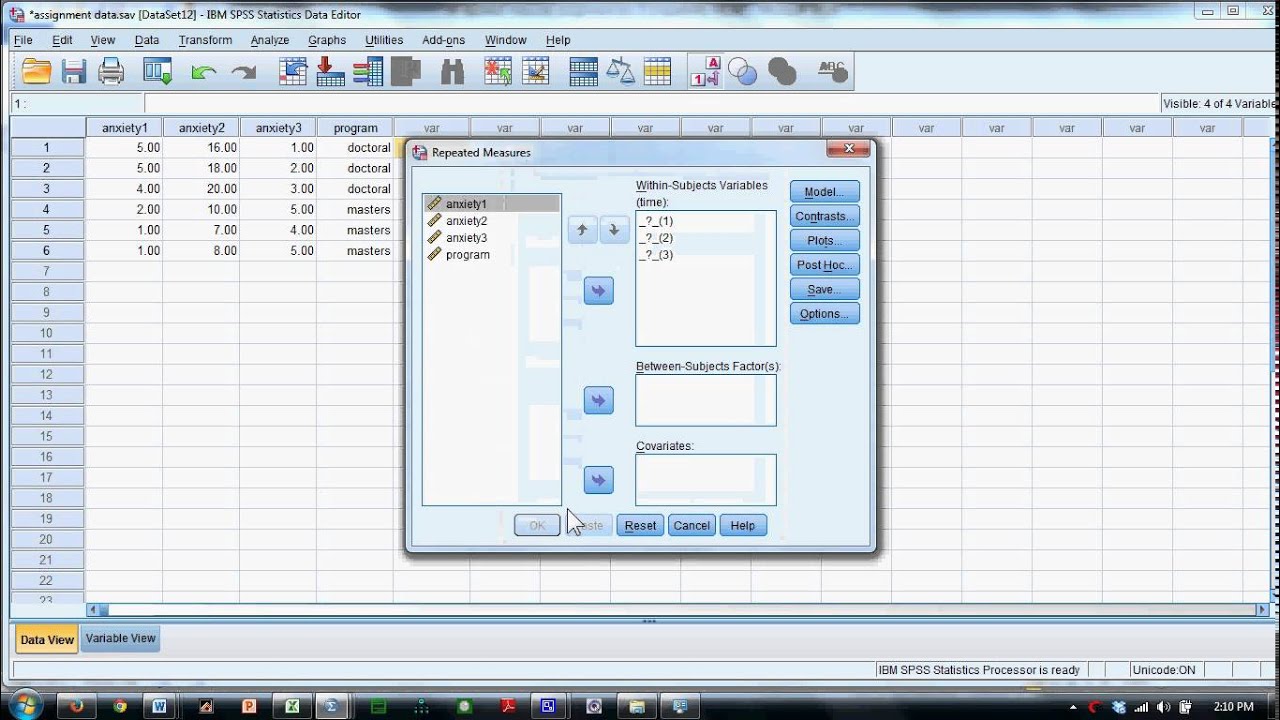

